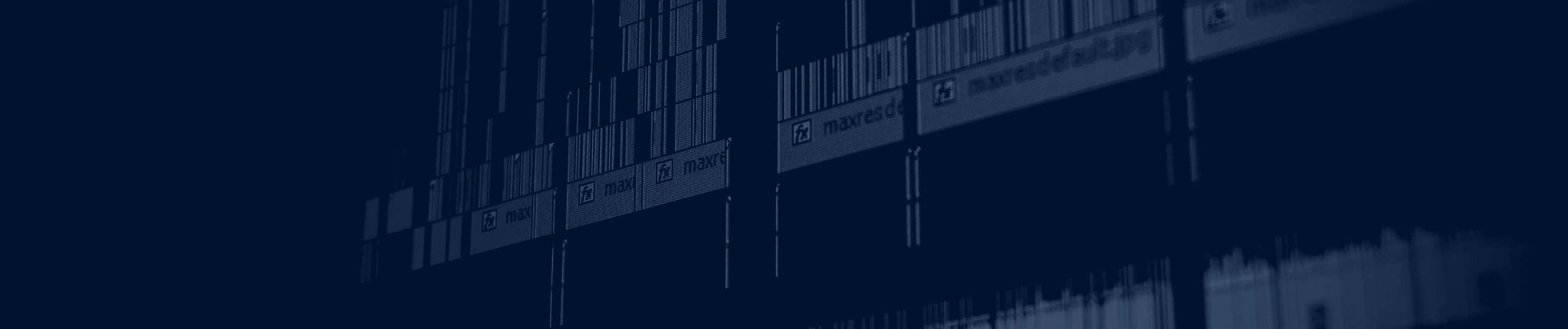
Syndicated Knowledge Base (KB) - Australia & NZ
crea.science has been using internet querying & scraping tools to collect publicly available information & insights on Australian Healthcare Professionals (HCPs), including 25 000+ General Practitioners (GPs), for more than 5 years. This information is captured and constantly updated & added to, in the crea.science Syndicated Knowledge Base.
What Information is Held in the Knowledge Base (KB)?
The KB holds a variety & depth of information on individual HCPs as well as their environment (i.e. medical practice). |
The information is extracted from many sources including directories, practice websites, medical publishing sites, social media sites, etc. It is stored in its raw form, e.g. clinical interests mentioned in biographies or as flags e.g. Y/N for Twitter account. |
The KB currently holds over 300 features and more are added as the needed information is identified. |
Updates are constantly performed to keep pace with HCPs evolving web profiles. |
How is the Knowledge Base Useful to Pharma?
The information held in the KB is traditionally used by Pharma in 3 ways.
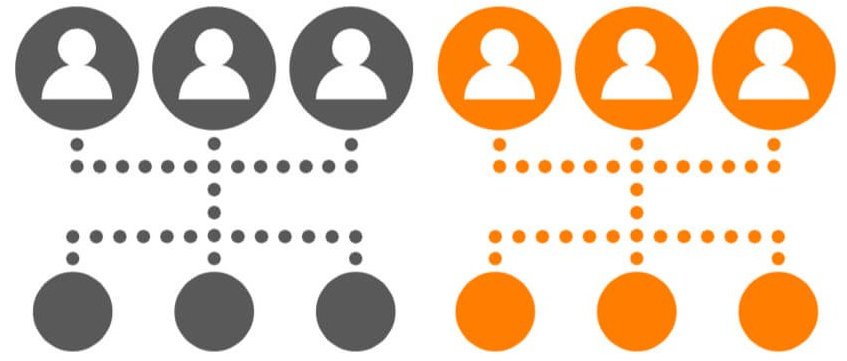
To Build HCP Profiles

To Build Composite HCP Indices

To Build HCP Indices via Extrapolation from Already Validated Reference Sets
Raw data are extracted as features or flags and used to build profiles e.g. stated clinical interests, use of social media, etc.
Machine Learning techniques are used to combine and investigate all the data and to generate indices e.g. for potential, influence, early adoption, proficiency.
The reference set is used to train the machine learning algorithm on what to look for in the data. Once validated, it may be used to predict potential, influence for all HCPs in the target population of interest.
Insights on GPs |
![]() |
Clinical Interests |
Social Media Usage |
Research Activities |
Online Self-Promotion |
Biography |
Quality of Care |
… |
Covers almost any publicly available information on the Internet |
Regular updates to keep pace with GPs evolving web profiles |
Insights on Practices |
![]() |
Use of Digital Technology: online bookings, digital content, blogs, website complexity |
Online Repeats |
Availability of Allied Health |
Business Hours, Bulk Billing |
Languages Spoken |
… |
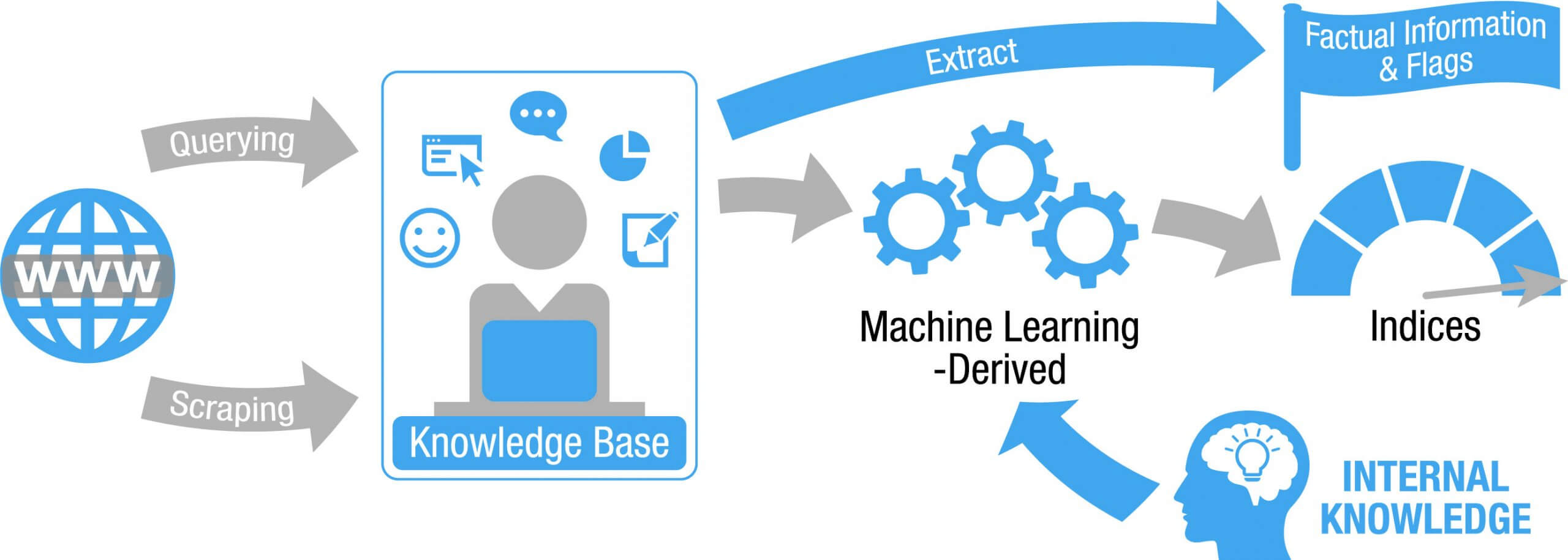
Where the client already has internal knowledge, e.g. a list of validated KOLs, the extracted data from the Knowledge Base (KB) for these already validated KOLs, can be used as a reference set to train the algorithm to identify other KOLs with similar profiles across all features in the KB. From a small set of known KOLs, all other HCPs can be assigned a potential “KOL Influence Score” based on their similarity to the reference set.”
If you possess existing data on some GPs’ digital engagement make use of our Knowledge Base and Machine Learning to expand predictions to all GPs in the target population.
Our Data Collection & Generation Process – So Much More Than Querying & Scraping
Web Querying |
A method of extracting information from the internet using keywords in a search engine, e.g. Google or MS Bing. |
Web Scraping | ![]() |
A method of extracting information from relevant websites. Scraping goes far beyond querying. Internet query may be used to identify websites for scraping. The depth of information scraped can include, Drs. interests, number in the practice, practice email, fax no., online bookings, bulk billing, car parking, etc. Large number of individual features or characteristics can be scraped in this manner. On their own some may seem irrelevant, but when combined and incorporated into the machine-learning algorithm, each feature and their complex relationships can add to the profile. |
Data Engineering – Extensive Data Validation & Cleansing | ![]() |
Over the years we have put in place complex data matching, duplicate identification, validation & cleansing algorithms to make sure that we correctly identify HCPs and that we extract relevant and consistent information.
Generating New Features | ![]() |
Using our knowledge of pharma & the business problem at hand, we also generate additional features based on the raw information extracted from queries & scraping results.
For research activities, we typically calculate the median authorship position for medical publications. The position in the list of authors may be used as a proxy for influence. We typically generate hundreds such features.
Machine Learning Based Data Aggregation | ![]() |
Typically results are aggregated into an index or score e.g. social networks – a higher score assigned where the search indicates high engagement with social networks.
The nature of the indices depends on the goal of the application, e.g. predicting potential, influence, early adoption, digital proficiency, digital engagement, etc.